Basic principles of radar object tracking
Radar object tracking technology plays a crucial role in many fields such as modern military, aerospace, and transportation. It can determine the real-time motion parameters of the object, such as position, velocity, acceleration, etc., providing key information for decision-making and control. With the continuous advancement of technology, radar object tracking technology is also constantly developing, gradually evolving from simple single object tracking in the early days to complex multi-object tracking, and achieving significant improvements in accuracy, reliability, and real-time performance.
Object tracking refers to the process and analysis of object position, velocity, and other information obtained by radar in a series of continuous observations, establishing a object motion trajectory model, and predicting the object’s state at future times. Its purpose is to accurately and real-time grasp the movement of the object, in order to make corresponding decisions and reactions. For example, in the field of military air defense, it is necessary to track enemy aircraft, missiles, and other objects to provide accurate object parameters for the launch of air defense weapons; In the field of transportation, it is used to monitor and manage aircraft, ships, vehicles, etc. to ensure traffic safety.
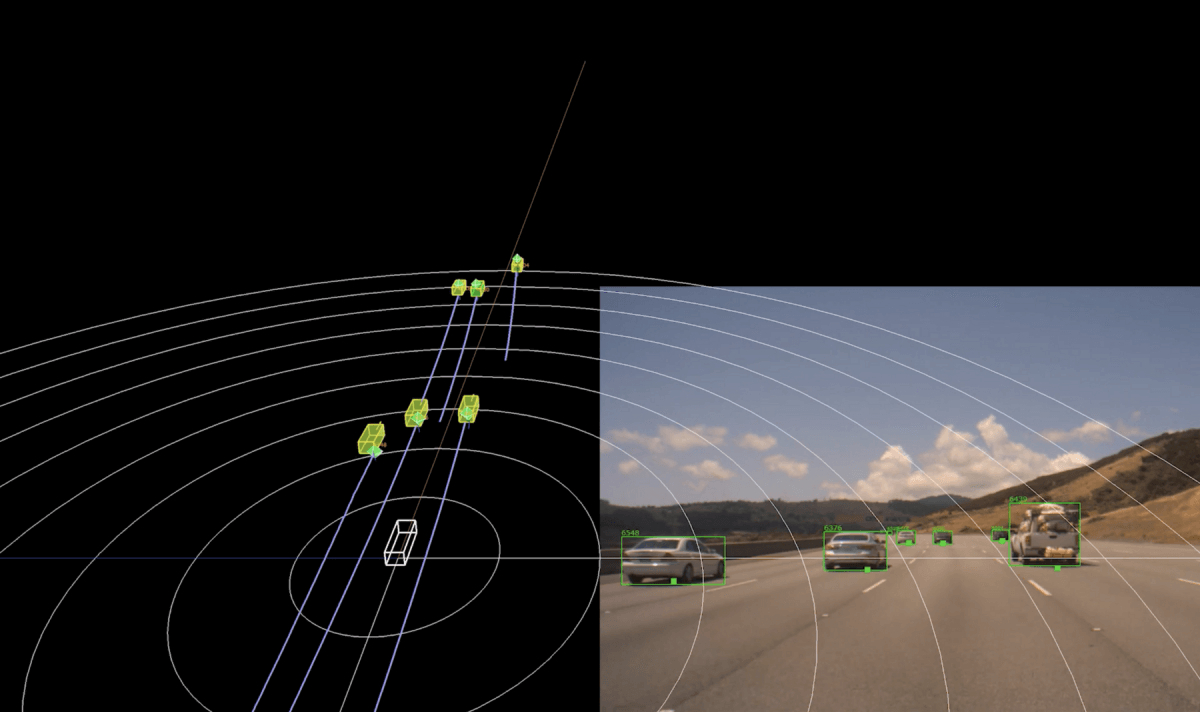
1. Data association algorithm for radar object tracking
Nearest Neighbor (NN) algorithm
Principle: In each scan, the current observed object echo is compared with the predicted object position from the previous moment, and the echo with the closest distance (usually measured by Euclidean distance or Mahalanobis distance) is selected as the observation associated with the object. For example, suppose there are three objectives A B、C, At the current moment, there are four observation points O1 O2、O3、O4, By calculating the distance between each predicted position of the object and the observation point, associate the observation point (such as O2) closest to the predicted position of object A with object A.
Advantages: Simple algorithm, low computational complexity, and easy implementation.
Disadvantage: When there are multiple objects and the objects are dense, it is easy to have false associations because it only considers the closest factor and does not take into account other information such as the motion trend of the objects. For example, in densely populated areas, observations belonging to object B may be incorrectly associated with object A.
Probabilistic Data Association (PDA) algorithm
Principle: Considering the presence of clutter and missed detections during the observation process, the PDA algorithm performs data association by calculating the correlation probability between each observation point and the object. It first calculates the probability that each observation point is a true object echo, which is based on the predicted position of the object and the distribution of observation points. For example, if there are multiple observation points at a certain moment, the PDA algorithm will predict the possible position range of the object based on its motion model, and then calculate the probability of each observation point appearing within this range to determine the degree of association between the observation point and the object.
Advantages: Compared to the nearest neighbor algorithm, it can better handle cluttered environments and improve the accuracy of data association.
Disadvantages: High computational complexity, especially when there are a large number of observation points, the computational workload will significantly increase. And it assumes that the observations of all objects are independent of each other, which may not fully match in actual complex scenarios.
Multiple Hypothesis Tracking (MHT) algorithm
Principle: The MHT algorithm establishes multiple possible trajectory hypotheses for each object. In each scan, all hypotheses are updated and evaluated based on new observational data. It considers various possible combinations between observed data and existing trajectory assumptions. For example, for a object, there may be multiple observation points that can be associated with it. The MHT algorithm will simultaneously preserve these possible associations and continuously update and filter hypotheses based on subsequent observations. By calculating the likelihood function of each hypothesis, determine which hypotheses are more likely to be the true object trajectory.
Advantages: It has strong ability to handle complex scenes, can effectively deal with object crossing, occlusion and other situations, and has high tracking accuracy.
Disadvantage: The computational workload is enormous, and the requirements for computing resources are extremely high. As the number of objects and observation points increases, the assumed number will grow exponentially, resulting in long computation time and difficulty in ensuring real-time performance.
2. Object Motion Model for Radar Object Tracking
Constant Velocity (CV) model for uniform linear motion
Principle: Assuming the object maintains uniform linear motion for a period of time. In a two-dimensional plane, the position of the object can be represented by coordinates (x, y), and the velocity can be represented by components (vx, vy). According to the kinematic formula, the position at time k+1 can be calculated based on the position and velocity at time k.
Advantages: The model is simple, the calculation is convenient, and it is suitable for scenarios where the object motion state is relatively stable and the speed changes are not significant, such as aircraft cruising in open airspace.
Disadvantage: When the object turns, accelerates, or decelerates, the model cannot accurately describe the object’s motion, which can lead to significant tracking errors.
Constant Acceleration (CA) Linear Motion Model
Principle: Considering that the object may have acceleration, an acceleration component is added to the CV model.
Advantages: It can better adapt to changes in object speed, and compared to the CV model, it has higher tracking accuracy when the object is accelerating or decelerating.
Disadvantage: When the acceleration of the object is not constant or complex maneuvers occur (such as curved flight), the descriptive ability of this model is limited.
Mobile object model
Singer model
Principle: This model assumes that the acceleration of the object is a random process, and the change in acceleration follows a first-order time-dependent Gaussian process with zero mean. By introducing an acceleration related time constant, the variation characteristics of acceleration can be described. In practical applications, it can handle object maneuvering situations well because it takes into account the randomness and variability of acceleration.
Advantages: Compared to CA models, it can track objects more accurately when they frequently maneuver. For example, when tracking a fighter jet, its maneuvering movements are complex, and the Singer model can predict the object’s position more accurately based on its acceleration characteristics.
Disadvantage: The selection of model parameters (such as acceleration related time constants) has a significant impact on tracking performance and needs to be adjusted reasonably according to specific object motion scenarios, otherwise it may lead to increased tracking errors.
Interactive Multiple Model (IMM)
Principle: The IMM model combines multiple different object motion models (such as CV model, CA model, Singer model, etc.). During the tracking process, each model calculates its own likelihood function based on the current observation data, and then switches to the model that best describes the current object motion state based on a certain probability through the interaction between models. For example, when the object is in a uniform straight-line flight phase, the likelihood function of the CV model is high, and the IMM model will have a higher probability of selecting the CV model for tracking; When the object begins to maneuver and turn, the likelihood function of the Singer model may increase, and the model will switch to the Singer model.
Advantages: It has strong adaptability and can provide high tracking accuracy in complex and changing object motion states. It fully utilizes the advantages of different models and dynamically selects the appropriate model based on the actual motion of the object.
Disadvantage: High computational complexity as multiple models need to run simultaneously and perform interactive calculations between them. Moreover, the selection of model sets and the determination of model switching probabilities also require certain experience and optimization.
3. Filtering algorithm for radar object tracking
Kalman Filter (KF)
Principle: Kalman filter is an optimal recursive filtering algorithm based on linear systems and Gaussian noise assumptions. It consists of two steps: prediction and update. In the prediction step, based on the motion model of the object, the current state is predicted using the estimated value of the previous state, and the covariance of the predicted state is calculated. For example, in a two-dimensional plane, based on the uniform linear motion model of the object, predict the current position and velocity using the previous position and velocity. In the update step, the predicted value is fused with the current observed value, and the Kalman gain is used to adjust the predicted value to obtain a more accurate state estimation. The Kalman gain is calculated based on the predicted covariance and observed noise covariance, reflecting the degree of influence of the observed values on state estimation.
Advantages: High computational efficiency, real-time processing of observation data, and good performance for object tracking in linear systems and Gaussian noise environments. In many practical applications, such as tracking aircraft during cruising, Kalman filtering can accurately estimate the position and velocity of objects.
Disadvantage: It requires the system to be linear and the noise to follow a Gaussian distribution. When there is nonlinearity or noise in the actual system that does not follow a Gaussian distribution, the performance of the Kalman filter will decrease and may even lead to filter divergence.
Extended Kalman Filter (EKF)
Principle: In order to handle nonlinear systems, EKF approximates the nonlinear function by performing a first-order Taylor expansion, and then applies the framework of Kalman filtering for processing. In the prediction step, a nonlinear motion model is used to predict the object state, and then the prediction function is linearized to calculate the Jacobian matrix. In the update step, the observation function is also linearized, the Jacobian matrix is calculated, and then the Kalman filter formula is used for state estimation and covariance update. For example, when tracking the orbit of a satellite, the motion equation of the satellite is nonlinear, and EKF can estimate the satellite state by linearizing the motion equation of the satellite.
Advantages: It can handle nonlinear systems to a certain extent and expand its application range compared to Kalman filtering. In many practical nonlinear system object tracking problems, such as attitude tracking of aircraft, EKF can provide relatively accurate state estimation.
Disadvantage: Due to the approximate linearization based on first-order Taylor expansion, when the nonlinearity of the system is high, the approximation error will increase, resulting in a decrease in filtering accuracy. Moreover, EKF is sensitive to the selection of initial values, and inappropriate initial values may affect the filtering effect.
Unscented Kalman Filter (UKF)
Principle: UKF does not approximate through linearization, but adopts a deterministic sampling strategy. It approximates the mean and covariance of the state well by selecting a set of Sigma points. In the prediction step, these Sigma points are propagated through a nonlinear motion model to obtain a new set of Sigma points, and then the mean and covariance of the predicted state are calculated based on these new Sigma points. In the update step, Sigma points are also propagated through a nonlinear observation model and the state is updated based on the observed values. For example, when tracking the motion of robots with complex nonlinear dynamics, UKF can use its sampling strategy to more accurately handle nonlinear problems.
Advantages: Compared to EKF, it has higher accuracy in dealing with nonlinear systems, especially suitable for systems with high nonlinearity. It avoids the errors caused by linearization approximation in EKF and can more accurately estimate the mean and covariance of the object state.
Disadvantage: The computational complexity is slightly larger than EKF, as it requires handling the propagation and computation of Sigma points. Moreover, the selection of Sigma points and the setting of related parameters can also affect the filtering performance.
Particle Filter (PF)
Principle: Particle filtering is based on Monte Carlo method, which represents the probability distribution of the object state through a large number of particles. In the initial stage, a set of particles is randomly generated, each with a corresponding weight, and the initial weights are usually set to be equal. In each iteration, the particle’s state is updated based on the object’s motion model, and then the weight of each particle is calculated based on the observed values. The weight reflects the degree of matching between the particle and the observed values. For example, if the predicted state of a particle is very close to the actual observed value, its weight will be relatively high. Finally, through the resampling process, particles with higher weights are retained and particles with lower weights are discarded to generate a new particle set for the next iteration.
Advantages: It has strong adaptability to nonlinear and non Gaussian systems, and can handle various complex object movements and observation models. In object tracking scenarios, such as tracking indoor moving objects, where the environmental noise is complex and non Gaussian, particle filtering can effectively track the object.
Disadvantages: The computational complexity increases with the number of particles, and the real-time performance is poor. Moreover, when the number of particles is insufficient, particle degradation may occur, where the weights of most particles become very small, and only a few particles contribute to state estimation, thereby affecting tracking accuracy.
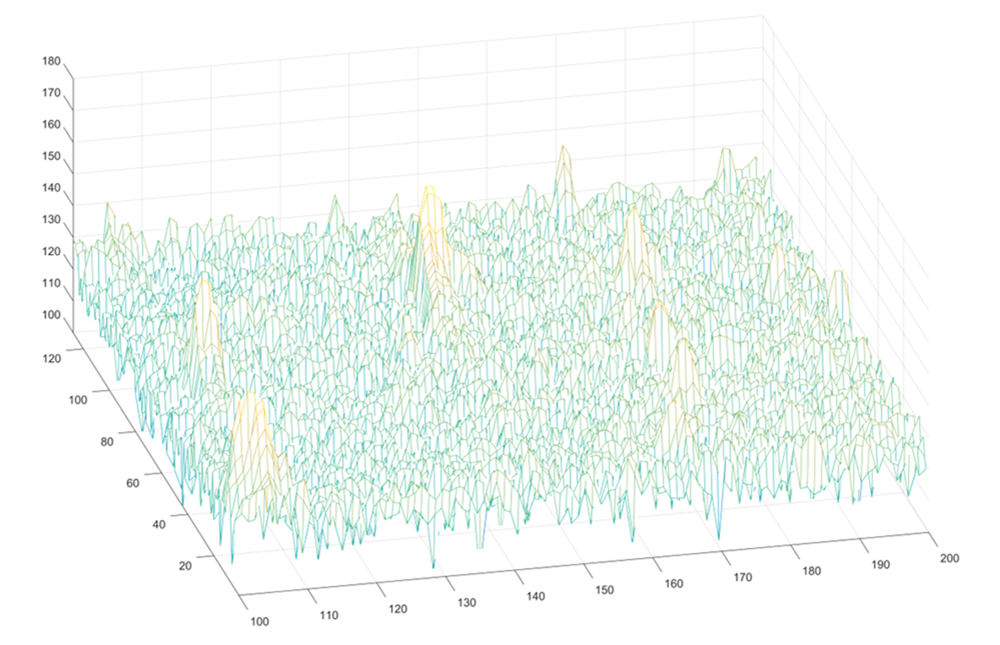
In intelligent transportation systems, radar object tracking technology is used to monitor vehicles on the road. By installing radar sensors on the roadside or vehicles, track the vehicle’s position, speed, acceleration, and other information. These information can be used for traffic flow monitoring, traffic signal control, and autonomous driving assistance systems. For example, in the autonomous vehicle, radar object tracking can monitor the moving state of the vehicle in front in real time, provide decision-making basis for the auto drive system, and realize automatic car following, obstacle avoidance and other functions.